Data Analysis and Visualization: A Comparison Before and After the Emergence of AI
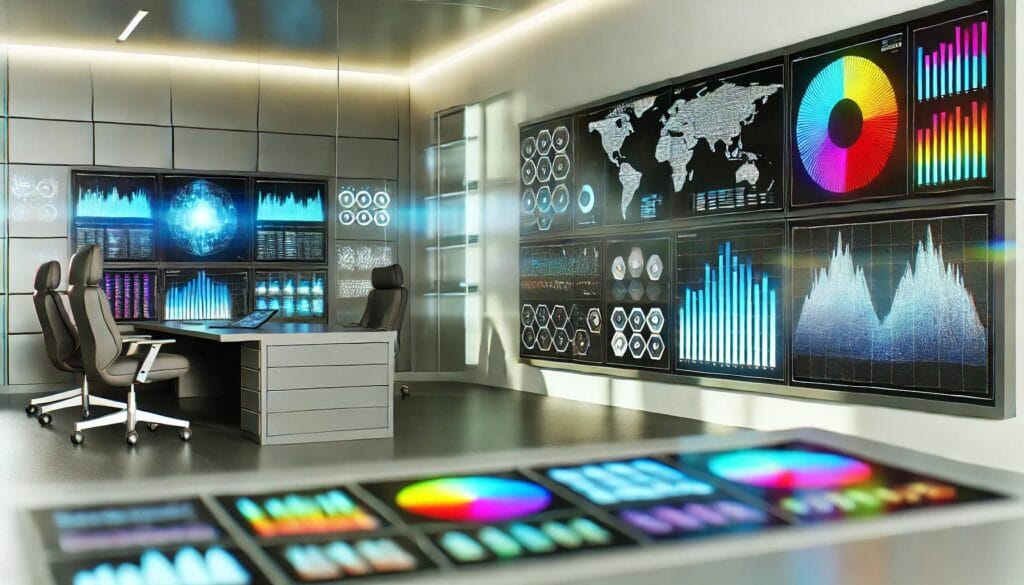
Data analysis and visualization have undergone profound transformations due to the development of artificial intelligence (AI). Before AI, these fields were largely dependent on manual processes, traditional statistical methods, and basic tools. Today, AI has revolutionized data analysis and visualization by enabling automation, enhanced predictive capabilities, and more insightful data representations.
Before AI: Traditional Methods
- Manual Data Collection and Cleaning:
- Before AI, data collection and cleaning were labor-intensive processes. Analysts had to manually sort through raw data, clean it for inconsistencies, and organize it into usable formats.
- The process was prone to human error and often resulted in inaccurate data, which could significantly impact the final analysis.
- Before AI, data collection and cleaning were labor-intensive processes. Analysts had to manually sort through raw data, clean it for inconsistencies, and organize it into usable formats.
- Statistical Analysis:
- Data analysis heavily relied on traditional statistical methods such as regression analysis, correlation, and hypothesis testing.
- These methods were useful but often limited in their ability to handle complex or large datasets. Analysts had to make assumptions, and the scope for deeper insights was constrained by the simplicity of models.
- Data analysis heavily relied on traditional statistical methods such as regression analysis, correlation, and hypothesis testing.
- Static Visualizations:
- Data visualization was limited to static charts and graphs such as bar charts, pie charts, and line graphs.
- Analysts manually created these visualizations using tools like Excel or basic statistical software, which lacked interactivity and real-time data analysis.
- Data visualization was limited to static charts and graphs such as bar charts, pie charts, and line graphs.
- Time-Consuming Processes:
- Most data analysis tasks were time-consuming and required significant human intervention.
- Generating insights from large datasets could take weeks or even months, especially for industries like healthcare, finance, and research.
- Most data analysis tasks were time-consuming and required significant human intervention.
After AI: The Transformational Impact
- Automated Data Cleaning and Preparation:
- AI has introduced powerful data preprocessing tools that can automatically clean, validate, and prepare datasets for analysis. Machine learning algorithms can now detect patterns and anomalies in data, reducing human error and speeding up the process.
- AI systems can handle vast amounts of unstructured data (e.g., text, images, sensor data), making it easier to extract meaningful insights.
- AI has introduced powerful data preprocessing tools that can automatically clean, validate, and prepare datasets for analysis. Machine learning algorithms can now detect patterns and anomalies in data, reducing human error and speeding up the process.
- Advanced Analytical Models:
- Machine learning algorithms, particularly deep learning and neural networks, have greatly expanded the scope of data analysis. AI can now identify complex patterns in large datasets that traditional methods would struggle to find.
- Predictive analytics has improved with AI, allowing businesses to forecast trends, behaviors, and outcomes with greater accuracy.
- Machine learning algorithms, particularly deep learning and neural networks, have greatly expanded the scope of data analysis. AI can now identify complex patterns in large datasets that traditional methods would struggle to find.
- Interactive and Real-Time Visualizations:
- AI-powered tools have enabled the creation of dynamic, interactive visualizations. These tools allow users to explore datasets in real-time, filtering and drilling down into the data for more granular insights.
- Technologies like augmented reality (AR) and virtual reality (VR) are even starting to make an impact on data visualization, offering 3D representations of complex datasets.
- AI-powered tools have enabled the creation of dynamic, interactive visualizations. These tools allow users to explore datasets in real-time, filtering and drilling down into the data for more granular insights.
- Faster Decision Making:
- AI has significantly reduced the time required to analyze data and generate insights. Automated systems can now provide immediate analysis and visualizations, allowing businesses and organizations to make data-driven decisions faster.
- Real-time data analysis is now possible with AI, enabling immediate responses to changing trends or emerging issues.
- AI has significantly reduced the time required to analyze data and generate insights. Automated systems can now provide immediate analysis and visualizations, allowing businesses and organizations to make data-driven decisions faster.
- Improved Accuracy and Objectivity:
- AI systems are trained to identify and learn from data without bias, making the analysis more objective and accurate. The likelihood of human error in analyzing and interpreting data has been greatly minimized.
- Machine learning models can evolve and adapt over time, improving the quality of insights as more data becomes available.
- AI systems are trained to identify and learn from data without bias, making the analysis more objective and accurate. The likelihood of human error in analyzing and interpreting data has been greatly minimized.
Key Differences in Data Analysis and Visualization Pre- and Post-AI
Aspect | Before AI | After AI |
---|---|---|
Data Collection & Cleaning | Manual, labor-intensive, prone to error | Automated, faster, AI-powered cleaning |
Analysis Methods | Traditional statistical methods | Advanced machine learning algorithms |
Visualization | Static charts, basic graphics | Interactive, real-time, immersive visualizations |
Speed | Slow, manual processes | Fast, automated, real-time analysis |
Accuracy | Prone to human error | High accuracy, reduces bias |
Scalability | Limited to smaller datasets | Handles massive datasets, unstructured data |
Conclusion
The advent of AI has brought about dramatic changes in the way data is analyzed and visualized. It has enabled the automation of tedious tasks, allowed for more advanced and accurate analysis, and created more interactive and real-time visualizations. As AI continues to evolve, its impact on data analysis and visualization will likely become even more profound, shaping the future of data-driven decision-making in various industries.
Source : Medium.com