Machine Learning & Artificial Intelligence: Revolutionizing the Future
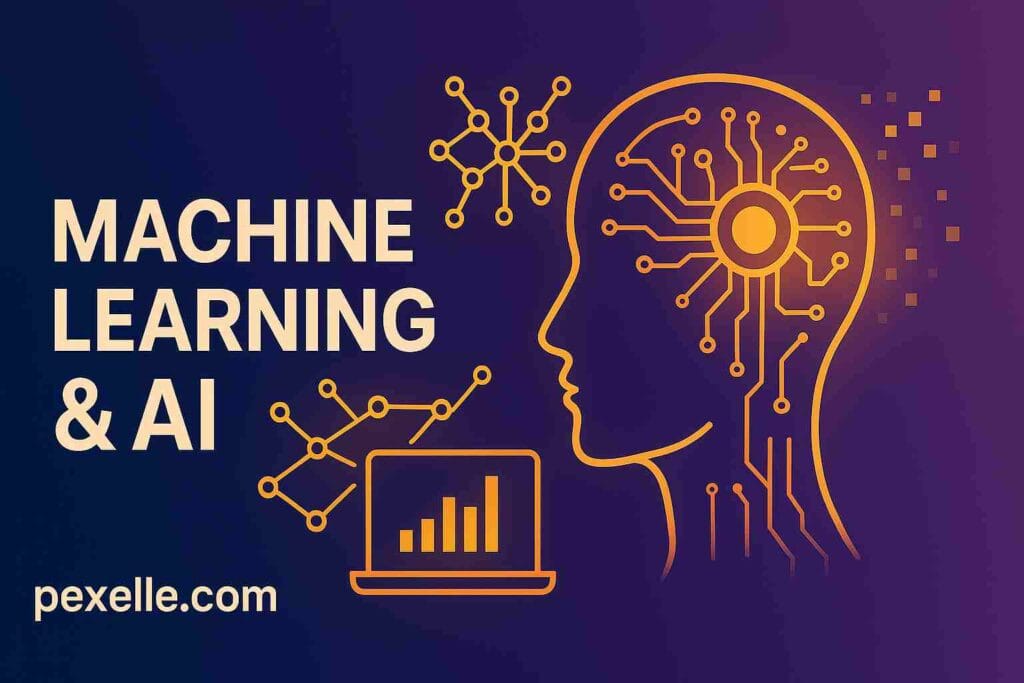
Introduction
Machine Learning (ML) and Artificial Intelligence (AI) are transforming industries and society at an unprecedented pace. These fields encompass a range of technologies that enable machines to learn from data, make decisions, and perform tasks traditionally requiring human intelligence. With applications in healthcare, finance, robotics, and more, ML and AI are driving significant advancements that are reshaping how we live and work.
What is Machine Learning?
Machine Learning is a subset of Artificial Intelligence that focuses on developing algorithms that allow computers to learn from and make predictions based on data. Unlike traditional programming, where every decision is explicitly coded, ML systems improve their performance over time as they are exposed to more data. The primary types of machine learning are:
- Supervised Learning: This is where the model is trained on a labeled dataset, meaning the algorithm learns from examples that are labeled with the correct answer. It is commonly used in classification and regression tasks.
- Unsupervised Learning: In this case, the model works with data that is not labeled. The goal is to identify patterns and structures in the data, often used in clustering and association tasks.
- Reinforcement Learning: Here, an agent learns by interacting with an environment and receiving feedback through rewards or penalties. It is widely used in robotics and gaming.
- Semi-Supervised and Self-Supervised Learning: These techniques combine elements of supervised and unsupervised learning to leverage both labeled and unlabeled data.
What is Artificial Intelligence?
Artificial Intelligence, on the other hand, is a broader concept that involves creating intelligent systems capable of performing tasks that would typically require human cognition. AI encompasses various subfields, including:
- Natural Language Processing (NLP): Enabling machines to understand and respond to human language, NLP powers technologies like chatbots and virtual assistants.
- Computer Vision: Allowing machines to interpret and make decisions based on visual data, it plays a critical role in facial recognition, autonomous vehicles, and medical imaging.
- Expert Systems: These are AI systems that simulate the decision-making ability of a human expert, commonly used in domains like medicine, finance, and engineering.
- Robotics: Integrating AI with physical machines to perform tasks autonomously, often used in manufacturing, healthcare, and logistics.
Applications of Machine Learning and AI
- Healthcare: AI is revolutionizing healthcare by aiding in diagnostics, drug discovery, and personalized treatment plans. Machine learning models can analyze medical images, predict disease progression, and even assist in surgery.
- Finance: AI-driven algorithms in the finance sector help with fraud detection, stock market prediction, and risk management. Machine learning models also power robo-advisors that provide investment recommendations.
- Transportation: AI is at the core of autonomous vehicles, where machine learning algorithms help self-driving cars navigate and make real-time decisions based on their environment.
- E-commerce and Marketing: AI-driven recommendation systems, chatbots, and personalized advertising are transforming the way businesses interact with customers, making marketing more efficient and targeted.
- Entertainment: Platforms like Netflix and Spotify use machine learning algorithms to provide personalized recommendations based on user preferences and behavior.
Challenges and Considerations
While AI and machine learning hold great potential, there are several challenges and considerations that need to be addressed:
- Data Privacy and Ethics: The use of personal data to train AI models raises significant privacy and ethical concerns. Proper safeguards and transparency are crucial to ensure that AI systems respect user privacy.
- Bias and Fairness: AI models can inherit biases present in the training data, leading to unfair or discriminatory outcomes. It’s essential to develop techniques to mitigate bias and ensure fairness in AI systems.
- Explainability: Many AI models, particularly deep learning networks, operate as “black boxes,” making it difficult to understand how they arrive at decisions. Efforts are underway to improve the transparency and explainability of AI systems.
- Job Displacement: The automation of tasks through AI and machine learning raises concerns about job displacement. However, there is also the potential for new job opportunities and industries to emerge.
The Future of AI and Machine Learning
The future of AI and machine learning holds exciting possibilities, with advancements in deep learning, quantum computing, and AI ethics likely to further enhance the capabilities of intelligent systems. As these technologies continue to evolve, their potential to solve complex global challenges, such as climate change, healthcare accessibility, and education, will be immense.
Conclusion
Machine learning and artificial intelligence are no longer futuristic concepts but are shaping the present and future of various industries. As these technologies evolve, they will continue to disrupt existing paradigms, offering new opportunities for innovation and progress. Embracing AI and ML responsibly will be key to maximizing their benefits while addressing the associated challenges.
Source : Medium.com